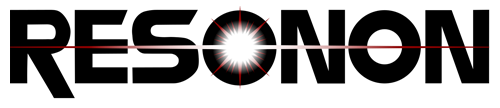
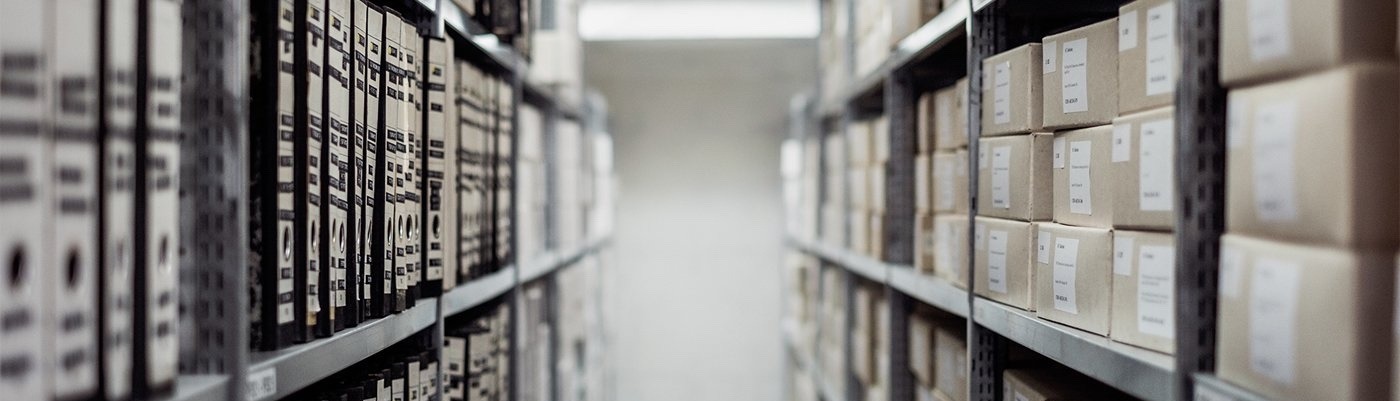
Research Publications
Publications
Below are research publications describing investigations using Resonon hyperspectral systems.
Let us know if you would like to list your publications here. Email us at inquiry@resonon.com or contact us here.
Biotechnology
Earth Sciences
Ecology
Environmental Monitoring
Food Science
Forensics
Industrial Applications
Physics & Engineering
Precision Agriculture: Crop Management
Precision Agriculture: Pest & Disease Management
Hyperspectral Imaging: General
Agronomy
Medical
Airborne Hyperspectral Remote Sensing
Agriculture & Food Technology
Institutional Partners
We are grateful for support from the following institutions:
Biotechnology
Henry Ndu, Akbar Sheikh-Akbari, Jiamei Deng, and Iosif Mporas, HyperVein: A Hyperspectral Image Dataset for Human Vein Detection, Sensors 24, (4) (2024).
Claretta J. Sullivan, Kennedy Brown, Chia‑Suei Hung, Joseph Kuo‑Hsiang Tang, Mark DeSimone, Vincent Chen, Pamela F. Lloyd, Maneesh Gupta, Abby Juhl, Wendy Crookes‑Goodson, Milana Vasudev, Patrick B. Dennis & Nancy Kelley‑Loughnane, Iridescent biofilms of Cellulophaga lytica are tunable platforms for scalable, ordered materials, Nature - Scientific Reports 13, 13192 (2023).
Robin S. Athawale, Ramdas D. Gore and Bharti W. Gawali, Hyperspectral Imaging for Seed Viability: A Review, Journal of Data Science and Intelligent Systems 00 (00), 1-12 (2023).
Yang Y, Nan R, Mi T, Song Y, Shi F, Liu X, Wang Y, Sun F, Xi Y, Zhang C, Rapid and Nondestructive Evaluation of Wheat Chlorophyll under Drought Stress Using Hyperspectral Imaging, International Journal of Molecular Sciences 24(6), 5825 (2023).
Vitor A. Gontijo da Cunha, Jeanette Hariharan, Yiannis Ampatzidis, Pamela D. Roberts, Early detection of tomato bacterial spot disease in transplant tomato seedlings utilising remote sensing and artificial intelligence, Biosystems Engineering 234, 172-186 (2023).
Ramy Abdlaty, Mohamed A. Abbass, and Ahmed M. Awadallah, Radiofrequency ablation for liver: Comparison between expert eye and hyperspectral imaging assessment, Photodiagnosis and Photodynamic Therapy 37, 102699 (2022).
Mohamed Hisham Fouad Aref, Mohamed A. Abbass, Abou-Bakr M. Youssef, Abdallah Abdelkader Hussein, Sara Abd El-Ghaffar, and Ramy Abdlaty, Optical Characterization of Biological Tissues in Visible and Near-Infrared Spectra, 13th International Conference on Electrical Engineering (ICEENG) , 9781827 (2022).
Ramy Abdlaty, Mohamed A. Abbass, Ahmed M. Awadallah, Radiofrequency ablation for liver: Comparison between expert eye and hyperspectral imaging assessment, Photodiagnosis and Photodynamic Therapy 37, 102699 (2022).
Abdlaty, R., Abbass, M.A. & Awadallah, A.M. , High Precision Monitoring of Radiofrequency Ablation for Liver Using Hyperspectral Imaging, Ann Biomed Eng 49, 2430–2440 (2021).
M. Guillemort, R. Midahuen, D. Archeny, C. Fulchiron, R. Montvernay, G. Perrin, and D. Leroux, Hyperspectral imaging for presumptive identification of bacterial colonies on solid chromogenic culture media, Proc. SPIE 9887, 98873L (2016).
D. Leroux, R. Midahuen, G. Perrin, J. Pescatore, and P. Imbaud, Hyperspectral imaging applied to microbial categorization in an automated microbiology workflow, Proc. SPIE-OSA 9537, 953726 (2015).
L. Polerecky, A. Bissett, M. Al-Najjar, P. Faerber, H. Osmers, P.A. Suci, P. Stoodley, and D. de Beer, Modular spectral imaging system for discrimination of pigments in cells and microbial communities, Appl. and Env. Microbio. 75, 758 (2009).
M. Kühl and L. Polerecky, Functional and structural imaging of phototrophic microbial communities and symbioses, Aquatic Microbial Ecology 53, 99 (2008).
A. Bachar, L. Polerecky, J.P. Fischer, K. Vamvakopoulos, D. de Beer, and H.M. Jonkers, Two-dimensional mapping of photopigment distribution and activity of Chloroflexus-like bacteria in a hypersaline microbi, FEMS Microbial Ecology 65, 434 (2008).
Earth Sciences
James Dillon, Christopher Donahue, Evan Schehrer, Karl Birkeland, and Kevin Hammonds , Mapping surface hoar from near-infrared texture in a laboratory, EGUsphere 2024, 1-36 (2024).
Zhaoxia Lou, Longzhe Quan, Deng Sun, Fulin Xia, Hailong Li, Zhiming Guo, Multimodal deep fusion model based on Transformer and multi-layer residuals for assessing the competitiveness of weeds in farmland ecosystems, International Journal of Applied Earth Observation and Geoinformation 127, 103681 (2024).
Yan, H., Zheng, R., Mallela, S., Russell, R., & Kursun, O. , Collection of a Hyperspectral Atmospheric Cloud Dataset and Enhancing Pixel Classification through Patch-Origin Embedding, Remote Sensing 16(17), 3315 (2024).
Mckenzie Skiles, C. Donahue, A. Hunsaker, J. Jacobs
, UAV hyperspectral imaging for multiscale assessment of Landsat 9 snow grain size and albedo, Frontiers in Remote Sensing 3, 1-16 (2023).
Alireza Sanaeifar, Ce Yang, Miguel de la Guardia, Wenkai Zhang, Xiaoli Li, Yong He, Proximal hyperspectral sensing of abiotic stresses in plants, Science of The Total Environment 861, 160652 (2023).
Ahed Alboody, Nicolas Vandenbroucke, Alice Porebski, Rosa Sawan, Florence Viudes, Perine Doyen, Rachid Amara, A New Remote Hyperspectral Imaging System Embedded on an Unmanned Aquatic Drone for the Detection and Identification of Floating Plastic Litter Using Machine Learning, Remote Sensing 15(14), 3455 (2023).
Wijayanti Nurul Khotimah, Mohammed Bennamoun, Farid Boussaid, Lian Xu, David Edwards, Ferdous Sohel , MCE-ST: Classifying crop stress using hyperspectral data with a multiscale conformer encoder and spectral-based tokens, International Journal of Applied Earth Observation and Geoinformation 118, 103286 (2023).
Sureka Thiruchittampalam, Simit Raval, Nancy Glenn, and Furqan Le-Hussain, Indirect remote sensing techniques for long term monitoring of CO2 leakage in geological carbon sequestration: A review, Journal of Natural Gas Science and Engineering 100, 104488 (2022).
Mohamed Karim El Oufir, Karem Chokmani , Anas El Alem, and Monique Bernier, Using Ensemble-Based Systems with Near-Infrared Hyperspectral Data to Estimate Seasonal Snowpack Density, Remote Sensing 14 no. 5, 1089 (2022).
Christopher Donahue, S. McKenzie Skiles, and Kevin Hammonds, Mapping liquid water content in snow at the millimeter scale: an intercomparison of mixed-phase optical property models using hyperspectral imaging and in situ measurements, The Cryosphere 16, 43-59 (2022).
Quanzhou Yu, Robert A. Mickler, Tianquan Liang, Yujie Liu, Jie Jiang, Kaishan Song, and Shaoqiang Wang, Hyperspectral differences between sunlit and shaded leaves in a Manchurian ash canopy in Northeast China, Remote Sensing Letters 13:8, 800-811 (2022).
Christopher Donahue and Kevin Hammonds
, Laboratory Observations of Preferential Flow Paths in Snow Using Upward-Looking Polarimetric Radar and Hyperspectral Imaging, Remote Sensing 14(10), 2297 (2022).
Sherry B. Hildreth, Evan S. Littleton, Leor C. Clark, Gabrielle C. Puller, Shihoko Kojima and
Brenda S. J. Winkel, Mutations that alter Arabidopsis flavonoid metabolism affect the circadian clock, The Plant Journal , 15718 (2022).
Hao Zhong, Wenshu Lin, Haoran Liu, Nan Ma, Kangkang Liu, Rongzhen Cao, Tiantian Wang, and Zhengzhao Ren, Identification of tree species based on the fusion of UAV hyperspectral image and LiDAR data in a coniferous and broad-leaved mixed forest in Northeast China, Frontiers in Plant Science 13, 1-17 (2022).
C. Donahue, S.M. Skiles, and K. Hammonds, In situ effective snow grain size mapping using a compact hyperspectral imager, Journal of Glaciology 68, 1-9 (2020).
L.H. Spangler et al, A shallow subsurface controlled release facility in Bozeman, Montana, USA, for testing near surface CO2 detection techni, Env. Earth Sci. 60, 227 (2010).
John Adler, A. Behar, & N. Jacobson, Airborne Hyperspectral Imaging of Supraglacial Lakes in Greenland's Ablation Zone, AGU Fall Meeting Abstracts , (2010).
Ecology
Benedict G. Hogan and Mary Caswell Stoddard
, Hyperspectral imaging in animal coloration research: A user-friendly pipeline for image generation, analysis, and integration with 3D modeling, PLOS Biology 22(12), e3002867 (2024).
Ting Zhang, Bo Tian, Yujue Wang, Dongyan Liu, Yunxuan Zhou, Daphne van der Wal, Mapping depth-integrated microphytobenthic biomass on an estuarine tidal flat using Sentinel satellite data, International Journal of Applied Earth Observation and Geoinformation 122, 103417 (2023).
Karin Kjernsmoa, Anna M. Lim, Rox Middleton, Joanna R. Hall, Leah M. Costello, Heather Whitney, Nicholas E. Scott-Samuel, and Innes C. Cuthill
, Beetle iridescence induces an avoidance response in naïve avian predators, Animal Behaviour, Volume 188, June 2022, Pages 45-50 188, 45-50 (2022).
Susannah M. Leahy, Dean R. Jerry, Brett B.C. Wedding, Julie B. Robins, Carole L. Wright, Aleksey Sadekov, Stephen Boyle, David B. Jones, Samuel M. Williams, Malcolm T. McCulloch, Steve Grauf, Luke Pavich, Mark McLennan, Michelle J. Sellin, Julie A. Goldsbury, Richard J. Saunders, Barramundi origins: Determining the contribution of stocking to the barramundi catch on Queensland’s east coast, Fisheries Research and Development Corporation 2018-047 Final Report , (2022).
Francisco Rodríguez Lorenzo, Miguel Placer Lorenzo, Luz Herrero Castilla, Juan Antonio Álvarez Rodríguez,
Sandra Iglesias, Santiago Gómez, Juan Manuel Fernández Montenegro, Estel Rueda, Rubén Diez-Montero,
Joan Garcia and Eva Gonzalez-Flo, Monitoring PHB production in Synechocystis sp. with hyperspectral images, Sater Science and Technology 86 No1, 211 (2022).
Marc Vermeulen, Diego Tamburini, Alicia C. McGeachy, Rebecca D. Meyers, Marc S. Walton, Multiscale characterization of shellfish purple and other organic colorants in 20th-century traditional enredos from Oaxaca, Mexico, Dyes and Pigments 206, 110663 (2022).
Yu R, Huo L, Huang H, Yuan Y, Gao B, Liu Y, Yu L, Li H, Yang L, Ren L, Luo Y, Early detection of pine wilt disease tree candidates using time-series of spectral signatures, Frontiers in Plant Science 13, 1000093 (2022).
R. Yu, Y. Luo, Q. Zhou, X. Zhang, D. Wu, and L. Ren, A machine learning algorithm to detect pine wilt disease using UAV-based hyperspectral imagery and LiDAR data at the tree level , Int. J. Appl. Ear. Obs. Geo. 101, 102363 (2021).
Run Yu, Lili Ren, and Youqing Luo, Early detection of pine wilt disease in Pinus tabuliformis in North China using a field portable spectrometer and UAV-based hyperspectral imagery, Forest Ecosystems 8, 44 (2021).
Run Yu, Youqing Luo, Haonan Li, Liyuan Yang, Huaguo Huang, Linfeng Yu, and Lili Ren, Three-Dimensional Convolutional Neural Network Model for Early Detection of PineWilt Disease Using UAV-Based Hyperspectral Images, Remote Sensing 13(20), 4065 (2021).
L. Ribeiro, A. Klock, J. Filho, M. Tramontin, A. Trapp, A. Mithofer, and C. Nansen, Hyperspectral imaging to characterize plant-plant communication in response to insect herbivory, Plant Methods 14:54, (2018).
Li X, Xu H, Feng L, Fu X, Zhang Y, Nansen C, Using proximal remote sensing in non-invasive phenotyping of invertebrates, PLoS One 12(5), e0176392 (2017).
P. Wilcox, T.M. Horton, E.Youn, M.K. Jeong, D. Tate, T. Herman, and C. Nansen, Evolutionary refinement approaches for band selection of hyperspectral images with applications to automatic monitoring , Intelligent Data Analysis 18, 25 (2014).
A. Chennu, P. Farber, N. Volkenborn, M.A.A. Al-Najjar, F. Janssen, D. deBeer, and L. Polerecky, Hyperspectral imaging of the microscale distribution and dynamics of microphytobenthos in intertidal sediments, Limnol. Oceanogr.: Methods 11, 511 (2013).
K. Barott, J. Smith, E. Dinsdale, M. Hatay, S. Sandin, and F. Rohwer, Hyperspectral and Physiological Analyses of Coral Algal Interactions, PloS ONE 4, e8043 (2009).
CJ Keith, KS Repasky, RL Lawrence, SC Jay, JL Carlsten, Monitoring effects of a controlled subsurface carbon dioxide release on vegetation using a hyperspectral imager, Int. J. of Greenhouse Gas Control 3, 626 (2009).
Environmental Monitoring
Pilar Aparicio-Rizzo, Dagoberto Poblete-Caballero, Cristian Vera-Bastidas, Iván Pérez-Santos, and Daniel Varela, Phytoplankton detection study through hyperspectral signals in Patagonian Fjords, EGU Sphere 2024, 3951 (2025).
Riley D. Logan and Joseph A. Shaw, Benthic river algae mapping using hyperspectral imagery from unoccupied aerial vehicles, J. Appl. Rem. Sens. 18(2), 024513 (2024).
Rajabi R, Zehtabian A, Singh KD, Tabatabaeenejad A, Ghamisi P and Homayouni S, Editorial: Hyperspectral imaging in environmental monitoring and analysis, Frontiers in Environmental Science 11, 1353447 (2024).
Hu, Hao, Hongkui Zhou, Kai Cao, Weidong Lou, Guangzhi Zhang, Qing Gu, and Jianhong Wang, Biomass Estimation of Milk Vetch Using UAV Hyperspectral Imagery and Machine Learning, Remote Sensing 16(12), 2183 (2024).
Waczak, John, Adam Aker, Lakitha O. H. Wijeratne, Shawhin Talebi, Ashen Fernando, Prabuddha M. H. Dewage, Mazhar Iqbal, Matthew Lary, David Schaefer, and David J. Lary, Characterizing Water Composition with an Autonomous Robotic Team Employing Comprehensive In Situ Sensing, Hyperspectral Imaging, Machine Learning, and Conformal Prediction, Remote Sensing 16(6), 996 (2024).
Veronika Kopačková-Strnadová, Martin Kýhos, Jan Jelének, Developing Scalable Monitoring System for Acid Mine Drainage Detection, 2024 IEEE International Geoscience and Remote Sensing Symposium IGARSS 2024, 3404-3408 (2024).
Naugle, M.S., Denis, H., Mocellin, V.J.L. et al., Heat tolerance varies considerably within a reef-building coral species on the Great Barrier Reef, Communications Earth & Environment 5(1), 525 (2024).
Mansurat A. Ali, Xueyan Lyu, Mahmut S. Ersan, Feng Xiao, Critical evaluation of hyperspectral imaging technology for detection and quantification of microplastics in soil, Journal of Hazardous Materials 476, 135041 (2024).
Kim T-H, Min JE, Lee HM, Kim KJ, Yang C-S, Application of Hyperspectral Image for Monitoring in Coastal Area with Deep Learning: A Case Study of Green Algae on Artificial Structure, Journal of Marine Science and Engineering 12(11), 2042 (2024).
MS Naugle, H Denis, VJL Mocellin, PW Laffy, I Popovic, LK Bay, EJ Howells, Environmental, host, and symbiont drivers of heat tolerance in a species complex of reef-building corals, Communications Earth & Environment 5, 252 (2024).
Liu, Bing and Tianhong Li, A Machine-Learning-Based Framework for Retrieving Water Quality Parameters in Urban Rivers Using UAV Hyperspectral Images, Remote Sensing 16(5), 905 (2024).
Krishna B Katuwal, Haoguang Yang, & Bingru Huang
, Evaluation of phenotypic and photosynthetic indices to detect water stress in perennial grass species using hyperspectral, multispectral and chlorophyll fluorescence imaging, Grass Research 3, 16 (2023).
Wang Huaiyu , Liu Yongmei , Ge Xinghua , Dong Xingzhi , Long Yongqing , Wang Lei, Discriminating Stellera chamaejasme in alpine grasslands using UAV hyperspectral imagery and multivariate analysis, Frontiers in Ecology and Evolution 11, Text (2023).
Byrd, Kristin B. ; Palacios, Sherry L. ; Taylor, Niky search by orcid ; Kokaly, Raymond F. ; Hoefen, Todd Michael ; Moskal, Stacy ; Woo, Isa ; De La Cruz, Susan , Hyperspectral remote sensing of intertidal microbial biofilm functional and taxonomic diversity, AGU Fall Meeting 2023 , 2023AGUFM.B21B..03B (2023).
Logan, Riley D., Madison A. Torrey, Rafael Feijó-Lima, Benjamin P. Colman, H. Maurice Valett, and Joseph A. Shaw, UAV-Based Hyperspectral Imaging for River Algae Pigment Estimation, Remote Sensing 15, 3148 (2023).
Shuyun Yuan, Ying Li, Fangwen Bao, Haoxiang Xu, Yuping Yang, Qiushi Yan, Shuqiao Zhong, Haoyang Yin, Jiajun Xu, Ziwei Huang, Jian Lin, Marine environmental monitoring with unmanned vehicle platforms: Present applications and future prospects, Science of the Total Environment 858(1), 159741 (2023).
Chao Chen, Yankun Chen, Haohai Jin, Li Chen, Zhisong Liu, Haozhe Sun, Junchi Hong, Haonan Wang, Shiyu Fang and Xin Zhang, 3D Model Construction and Ecological Environment Investigation on a
Regional Scale Using UAV Remote Sensing, Intelligent Automation & Soft Computing , Full Text (2023).
Gao, B.; Yu, L.; Ren, L.; Zhan, Z.; Luo, Y. , Early Detection of Dendroctonus valens Infestation at Tree Level with a Hyperspectral UAV Image, Remote Sensing 15(2), 407 (2023).
Zhou, Quan, Linfeng Yu, Xudong Zhang, Yujie Liu, Zhongyi Zhan, Lili Ren, and Youqing Luo, Fusion of UAV Hyperspectral Imaging and LiDAR for the Early Detection of EAB Stress in Ash and a New EAB Detection Index—NDVI(776,678), Remote Sensing 14 no. 10 , 2428 (2022).
Jonathan Teague, David A. Megson-Smith, Michael J. Allen, John C.C. Day, and Thomas B. Scott
, A Review of Current and New Optical Techniques for Coral Monitoring, Oceans 3(1), 30-45 (2022).
Joseph Michael Odhiambo, Dr. Mgala Mvurya, Dr. Anthony Luvanda, Dr. Fullgence Mwakondo, Deep Learning Algorithm for Identifying Microplastics in Open Sewer Systems: A Systematic Review, The International Journal of Engineering and Science 11-5, 11-18 (2022).
Liang Xinlian, Kukko Antero, Balenovic Ivan, Saarinen Ninni, Junttila Samuli, Kankare Ville, Holopainen Markus, Mokroš Martin, Surový Peter, Kaartinen Luka, Honkavaara Eija, Näsi Jingbin, Hollaus Markus, Tian Jiaojiao, Yu Xiaowei, Pan Jie, Cai Shangshu, Virtanen Juho-Pekk, Wang Yunsheng, Hyyppä Juha, Close-Range Remote Sensing of Forests: The state of the art, challenges, and opportunities for systems and data acquisitions, IEEE Geoscience and Remote Sensing Magazine 10-3, 32-71 (2022).
Zhou Quan, Linfeng Yu, Xudong Zhang, Yujie Liu, Zhongyi Zhan, Lili Ren, and Youqing Luo, Fusion of UAV Hyperspectral Imaging and LiDAR for the Early Detection of EAB Stress in Ash and a New EAB Detection Index—NDVI(776,678), Remote Sensing 14(10), 2428 (2022).
Michael S. Watt, Tomás Poblete, Dilshan de Silva, Honey Jane C. Estarija, Robin J.L. Hartley, Ellen Mae C. Leonardo, Peter Massam, Henning,Buddenbaum, Pablo J. Zarco-Tejada, Prediction of the severity of Dothistroma needle blight in radiata pine using plant based traits and narrow band indices derived from UAV hyperspectral imagery, Preprint Posted 28 Nov 2022, Text (2022).
D.J. Lary, D. Schaefer, J. Waczak, A. Aker, A. Barbosa, L.O.H. Wijeratne, S. Talebi, B. Fernando, J. Sadler, T. Lary and M..D. Lary, Autonomous Learning of New Environments with a Robotic Team Employing Hyper-Spectral Remote Sensing, Comprehensive In-Situ Sensing and Machine Learning, Sensors 21, 2240 (2021).
H. Qin, W. Zhou, Y. Yao, and W. Wang, Estimating Aboveground Carbon Stock at the Scale of Individual Trees in Subtropical Forests Using UAV LiDAR and Hyperspectral Data, Rem. Sens. 13, 4969 (2021).
Chunmao Zhu, Yugo Kanaya, Masashi Tsuchiya, Ryota Nakajima, Hidetaka Nomaki, Tomo Kitahashi, Katsunori Fujikura, Optimization of a hyperspectral imaging system for rapid detection of microplastics down to 100 µm, MethodsX 8, 101175 (2021).
Faltynkova, A., Johnsen, G. & Wagner, Hyperspectral imaging as an emerging tool to analyze microplastics: A systematic review and recommendations for future development, Microplastics and Nanoplastics 1, 13 (2021).
W. Pi, Y. Bi, J. Du, H. Yang, X Zhang, and Y. Kang, Classification of Grassland Desertification in China Based on Vis-NIR UAV Hyperspectral Remote Sensing, Spectroscopy Online 35, 39-50 , (2020).
N.V. Scott and J. McCarthy, Potassium Phosphorus Nitrate Detection and Spectral Segmentation Using Polarized Hyperspectral Imagery in High Reflective Glare Environments, Presentation at 9th Annual Forensic Science Symposium , June (2020).
C. Zhu, YU. Kanaya, R. Nakajima, M. Tsuchiya, H. Nomaki, T. Kitahashi, and K. Fujikura, Characterization of microplastics on filter substrates based on hyperspectral imaging: Laboratory assessments, Environmental Pollution 263, 114296 (2020).
Y Gai, D Yu, Y Zhou, L Yang, C Chen, J Chen, An Improved Model for Chlorophyll-a Concentration Retrieval in Coastal Waters Based on UAV-Borne Hyperspectral Imagery: A Case Study in Qingdao, China., Water 12(10), 2769 (2020).
J. Lekki, S. Ruberg, C. Binding, R. Anderson, and A. VanderWoude, Airborne hyperspectral and satellite imaging of harmful algal blooms in the Great Lakes Region: Successes in sensing algal blooms, J. Great Lakes Research 45, 405 (2019).
C. Zhihu, T. Qiangqian, Z. Zeying, and Y. Yanbin, Research on hyperspectral remote sensing image acquisition and processing technology based on uav, Journal of Guizhou Normal University (Natural Sciences) 37, 52-57 (2019).
Ricardo Flores & Hector Loro, Analysis of contamination of soils by petroleum hydrocarbons using hyperspectral images in the NIR, XVIII Meeting of physics , (2019).
W. Castro, J. Oblitas, J. Maicelo, and H. Avila-George, Evaluation of Expert Systems Techniques for Classifying Different Stages of Coffee Rust Infection in Hyperspectral Images, Int. J. Comput. Intell. Sys. 11, 86-100 (2018).
N. Scott and I. Moore, Nonnegative matrix factorization based feature selection analysis for hyperspectral imagery of sediment-laden riverine flow, Proc. SPIE 10631, 2301273 (2018).
C. McCann, K.S. Repasky, R. Lawrence, and S. Powell, Multi-temporal mesoscale hyperspectral data of mixed agricultural and grassland regions for anomaly detection, ISPRS Journal of Photogrammetry and Remote Sensing 131, 121 (2017).
Chennu, A., Färber, P., De’ath, G. et al., A diver-operated hyperspectral imaging and topographic surveying system for automated mapping of benthic habitats, Scientific Reports 7, 7122 (2017).
R. Hruska, J. Mitchell, M. Anderson and N.F. Glenn, Radiometric and Geometric Analysis of Hyperspectral Imagery Acquired from an Unmanned Aerial Vehicle, Remote Sensing 4, 2736 (2012).
M.C.L. Patterson and A. Brescia, Operation of small sensor payloads on tactical sized unmanned air vehicles, Aeronautical Journal 114, 427 (2010).
R.C. Swanson, M.R. Kehoe, C.W. Smith, T.S. Moon, R. Bousquet, S.W. Brown, K.R. Lykke, P. Maciejewski, and K. Barnard, Compact Anamorphic Imaging Spectrometer, 2007 Meeting of the Military Sensing Symposia (MSS) Speciality Group On Camouflage, Concealment & Deception 1, (2007).
Food Science
Williams L, Shukla P, Sheikh-Akbari A, Mahroughi S, Mporas I., Measuring the Level of Aflatoxin Infection in Pistachio Nuts by Applying Machine Learning Techniques to Hyperspectral Images, Sensors (Basel) 25(5), 1548 (2025).
Md Wadud Ahmed, Asher Sprigler, Jason Lee Emmert, Ryan N Dilger, Girish Chowdhary, Mohammed Kamruzzaman, Non-destructive detection of pre-incubated chicken egg fertility using hyperspectral imaging and machine learning, Smart Agricultural Technology 10, 100857 (2025).
Vera, W., Avila-George, H., Mogollón, J. et al., Food fraud detection in Octopus mimus using hyperspectral imaging and machine learning techniques, Neural Computing and Applications 37, 2369–2381 (2025).
Yang, Y., Wijewardane, N.K., Harvey, L. et al., Sweetpotato moisture content and textural property estimation using hyperspectral imaging and machine learning, Food Measure 19, 2700–2716 (2025).
Naila Kanwal, Wiebke Kämper, Michael B. Farrar, Mahshid Tootoonchy, Clayton Lynch, Joel Nichols, Helen M. Wallace, Stephen J. Trueman, Shahla Hosseini Bai, Rapid assessment of lychee and mango fruit quality using hyperspectral imaging, LWT 224, 117833 (2025).
E. Fulladosa, M.W.S. Chong, A.J. Parrott, R. dos Santos, J. Russell, and A. Nordon, Developing robust food composition models: Strategies for handling temperature and packaging variations in dry-cured ham using near infrared spectrometry, Spectrochimica Acta Part A: Molecular and Biomolecular Spectroscopy 332, 125823 (2025).
Mateo, F.; Mateo, E.M.; Tarazona, A.; García-Esparza, M.Á.; Soria, J.M.; Jiménez, M. , New Strategies and Artificial Intelligence Methods for the Mitigation of Toxigenic Fungi and Mycotoxins in Foods, Toxins 17(5), 231 (2025).
Yue Sun, Nikhita Sai Nayani, Yixiang Xu, Zhanfeng Xu, Jun Yang, and Yiming Feng, Rapid and Nondestructive Determination of Oil Content and Distribution of Potato Chips Using Hyperspectral Imaging and Chemometrics, ACS Food Science & Technology 4 (6), 1579-1588 (2024).
Tsvakai Gama, Michael B. Farrar, Mahshid Tootoonchy, Helen M. Wallace, Stephen J. Trueman, Iman Tahmasbian, Shahla Hosseini Bai, Hyperspectral imaging predicts free fatty acid levels, peroxide values, and linoleic acid and oleic acid concentrations in tree nut kernels, LWT 199, 116068 (2024).
Rajendra Hamad, Subir Kumar Chakraborty, Adinath Kate, Shekh Mukhtar Mansuri, Understanding the changes in millable oil content and fatty acid composition of infrared radiation treated mustard (Brassica juncea) seeds using Vis-NIR-SWIR hyperspectral imaging, Infrared Physics & Technology 137, 105128 (2024).
Md. Toukir Ahmed, Md Wadud Ahmed, Ocean Monjur, Jason Lee Emmert, Girish Chowdhary, Mohammed Kamruzzaman, Hyperspectral Image Reconstruction for Predicting Chick Embryo Mortality Towards Advancing Egg and Hatchery Industry, Smart Agricultural Technology 9, 100533 (2024).
Michael B. Farrar, Reza Omidvar, Joel Nichols, Daniele Pelliccia, Suhad Lateef Al-Khafaji, Iman Tahmasbian, Nimanie Hapuarachchi, Shahla Hosseini Bai, Hyperspectral imaging predicts macadamia nut-in-shell and kernel moisture using machine vision and learning tools, Computers and Electronics in Agriculture 224, 109209 (2024).
S. Md. Mansoor Roomi, B. Sathya Bama, V. Puvi Lakshmi, M. Vaishnavi, Hyperspectral dataset of pure and pesticide-coated apples for measuring the level of fertilizers used, Data in Brief 49, 109321 (2023).
Irene Teixido-Orries, Francisco Molino, Ferran Gatius, Vicente Sanchis, Sonia Marín, Near-infrared hyperspectral imaging as a novel approach for T-2 and HT-2 toxins estimation in oat samples, Food Control 153, 109952 (2023).
Sai Xu, Huazhong Lu, Changxiang Fan, Guangjun Qiu, Christopher Ference,
Xin Liang, Jian Peng, Visible and near-infrared hyperspectral imaging as an intelligent tool for parasite detection in sashimi, LWT 181, 114747 (2023).
Irene Teixido-Orries, Francisco Molino, Antoni Femenias, Antonio J. Ramos, Sonia Marín, Quantification and classification of deoxynivalenol-contaminated oat samples by near-infrared hyperspectral imaging, Food Chemistry 417, 135924 (2023).
YJ Davur, W Kämper, K Khoshelham, SJ Trueman, SH Bai, Estimating the Ripeness of Hass Avocado Fruit Using Deep Learning with Hyperspectral Imaging, Horticulturae 9(5), 599 599 (2023).
S. Karthika Shree; Vaishali Vijayarajan; B. Sathya Bama; S. Mohammed Mansoor Roomi, Milk Quality Inspection Using Hyperspectral Imaging, 2023 International Conference on Signal Processing, Computation, Electronics, Power and Telecommunication (IConSCEPT) , 1-6 (2023).
Piyush Pandey, Patrick Veazie, Brian Whipker, Sierra Young, Predicting foliar nutrient concentrations and nutrient deficiencies of hydroponic lettuce using hyperspectral imaging, Biosystems Engineering 230, 458-469 (2023).
Irene Teixido-Orries, Francisco Molino, Antoni Femenias, Antonio J. Ramos, Sonia Marín, Quantification and classification of deoxynivalenol-contaminated oat samples by near-infrared hyperspectral imaging, Food Chemistry 417, 135924 (2023).
Dhakal, K.; Sivaramakrishnan, U.; Zhang, X.; Belay, K.; Oakes, J.; Wei, X.; Li, S. , Machine learning analysis of hyperspectral images of damaged wheat kernels
, Sensors 23, 3523 (2023).
Abebe, A.M.; Kim, Y.; Kim, J.; Kim, S.L.; Baek, J., Image-Based High-Throughput Phenotyping in Horticultural Crops, Plants 12(10), 2061 (2023).
Azfar Ismail, Dong‑Gyun Yim, Ghiseok Kim, Cheorun Jo, Hyperspectral imaging coupled with multivariate analyses for efficient prediction of chemical, biological and physical properties of seafood products
, Food Engineering Reviews 15, 41-55 (2023).
Yifei Han, Shahla Hosseini Bai, Stephen J. Trueman, Kourosh Khoshelham & Wiebke Kämper, Predicting the ripening time of ‘Hass’ and ‘Shepard’ avocado fruit by hyperspectral imaging, Precision Agriculture 24, 1889-1905 (20223).
Abdulridha J, Ampatzidis Y, Qureshi J, and Roberts P, Identification and Classification of Downy Mildew Severity Stages in Watermelon Utilizing Aerial and Ground Remote Sensing and Machine Learning
, Frontiers in Plant Science , 20 May (2022).
Shubhangi Srivastava and Hari Niwas Mishra, Detection of insect damaged rice grains using visible and near infrared hyperspectral imaging technique, Chemometrics and Intelligent Laboratory Systems 221, 104489 (2022).
Shengnan Wang, Yong Tan, Chunyu Liu, Shaozhong Song, Zheng Li, Variety Classification and Identification of Soybean Seeds Based on Hyperspectral Imaging Method, Journal of Sensor Technology and Application 10(2), 177-186 (2022).
Wenyang Jia, Saskia van Ruth, Nigel Scollan, and Anastasios Koidis, Hyperspectral Imaging (HSI) for meat quality evaluation across the supply chain: Current and future trends, Current Research in Food Science 5, 1017-1027 (2022).
Hongyu Liu, Fuheng Qu, Yong Yang, Wanting Li, and Zhonglin Hao, Soybean Variety Identification Based on Improved ResNet18 Hyperspectral Image, Journal of Physics: Conference Series 2284, 012017 (2022).
Xiang Yun, Chen Qijun, Su Zhongjing, Zhang Lu, Chen Zuohui, Zhou Guozhi, Yao Zhuping, Xuan Qi, and Cheng Yuan, Deep Learning and Hyperspectral Images Based Tomato Soluble Solids Content and Firmness Estimation, Frontiers in Plant Science 13, 860656 (2022).
Eshkabilov, Sulaymon, John Stenger, Elizabeth N. Knutson, Erdem Küçüktopcu, Halis Simsek, and Chiwon W. Lee, Hyperspectral Image Data and Waveband Indexing Methods to Estimate Nutrient Concentration on Lettuce (Lactuca sativa L.) Cultivars, Sensors 22(21), 8158 (2022).
I. Tahmasbian, N.K. Morgan, S.H. Bai , M.W. Dunlop, and A.F. Moss, Comparison of Hyperspectral Imaging and Near-Infrared Spectroscopy to Determine Nitrogen and Carbon Concentrations in Wheat, Remote Sens. 13, 1128 (2021).
Y. Wei, X. Li, X. Pan, and L. Li, Nondestructive Classification of Soybean Seed Varieties by Hyperspectral Imaging and Ensemble Machine Learning Algorithms, Sensors 20, 6980 (2020).
M.A. Faqeerzada, M. Perez, S. Lohumi, H. Lee, G. Kim, C. Wakholi, R. Joshi, and B.K. Cho, Online Application of a Hyperspectral Imaging System for the Sorting of Adulterated Almonds, Applied Sciences 10, 6569 (2020).
S. Gutierrez, J. Tardaguila, J. Fernandez-Novales and M.P. Diago, On-the-go hyperspectral imaging for the in-field estimation of grape berry soluble solids and anthocyanin concentration, Aust. J. Grape Wine Res. 18, 173-182 (2018).
N. Vasquez a, C. Magan, J. Oblitas, T. Chuquizuta, H. Avila-George, and W. Castro, Comparison between artificial neural network and partial least squares regression models for hardness modeling during the ripening
process of Swiss-type cheese using spectral profiles, J. Food E.ng. 219, 8-15 (2018).
W. Castro, J.M. Prieto, R. Guerra, T. Chuquizuta, W.T. Medina, B. Acevedo-Juarez, and H. Avila-George, Feasibility of using spectral profiles for modeling water activity in five varieties of white quinoa grains, J. Food Eng. 238, 95-102 (2018).
M. Matzrafi, I. Herrmann, C. Nansen, T. Kliper, Y. Zait, T. Ignat, D. Siso, B. Rubin, A. Karnieli, and H. Eizenberg, Hyperspectral Technologies for Assessing Seed Germination and Trifloxysulfuron-methyl Response in Amaranthus palmeri, Frontiers in Plant Science 8, 474 (2017).
V. Aredo, L. Velásquez, and R. Siche
, Prediction of beef marbling using Hyperspectral Imaging (HSI) and Partial Least Squares Regression (PLSR), Scientia Agropecuaria 8(2), 169-174 (2017).
C Nansen, G. Zhao, N. Dakin, C. Zhao, and S.R. Turner, Using hyperspectral imaging to determine germination of native Australian plant seeds, Journal of Photochemistry & Photobiology B 145, 19 (2015).
C. Nansen, T. Herrman, and R. Swanson, Machine Vision Detection of Bonemeal in Animal Feed Samples, Applied Spectroscopy 64, 637 (2010).
C. Nansen, M. Kolomeits, and X. Gao, Considerations regarding the use of hyperspectral imaging data in classifications of food products, exemplified by analy, J. of Ag. and Food Chem. 14, 2933 (2008).
Forensics
Ana Belén López-Baldomero, Marco Buzzelli, Francisco Moronta-Montero, Miguel Ángel Martínez-Domingo, Eva María Valero, Ink classification in historical documents using hyperspectral imaging and machine learning methods, Spectrochimica Acta Part A: Molecular and Biomolecular Spectroscopy 335, 125916 (2025).
Jesse Casana and Carolin Ferwerda, Drone-Acquired Short-Wave Infrared (SWIR) Imagery in Landscape Archaeology: An Experimental Approach, Remote Sensing 16 (10), 1671 1671 (2024).
Miriam Medina-García, Esteban A. Roca-Nasser, Miguel A. Martínez-Domingo, Eva M Valero, Alejandra Arroyo-Cerezo, Luis Cuadros-Rodríguez, Ana M. Jiménez-Carvelo, Towards the establishment of a green and sustainable analytical methodology for hyperspectral imaging-based authentication of wholemeal bread, Food Control 166, 110715 (2024).
Eva M. Valero, Miguel A. Martínez-Domingo, Ana B. López-Baldomero, Ana López-Montes, David Abad-Muñoz, José L. Vílchez-Quero, Unmixing and pigment identification using visible and short-wavelength infrared: Reflectance vs logarithm reflectance hyperspaces, Journal of Cultural Heritage 64, 290-300 (2023).
Marc Vermeulen, Annette S. Ortiz Miranda, Diego Tamburini, Sol E. Rivera Delgado, and Marc Walton, A multi-analytical study of the palette of impressionist and post-impressionist Puerto Rican artists
, Heritage Science 10, 44 (2022).
N.V. Scott and S. Jensen, Blood Stain Detection on Camouflage Clothing Using Machine Learning Analysis of Noisy Hyperspectral Imagery, Presentation at 9th Annual Forensic Science Symposium , June (2020).
J. Striova, A. Dal Fovo, and R. Fontana, Reflectance imaging spectroscopy in heritage science, La Rivista del Nuovo Cimento 43, 515 (2020).
M.Á. Martínez-Domingo, A.I. Castillo, E.V. García, and E.M. Valero, Evaluation of Cleaning Processes Using Colorimetric and Spectral Data for the Removal of Layers of Limewash from Medieval Plasterwork, Sensors 20, 7147 (2020).
M.A. Martinez, E.M. Valero, J.L. Nieves, R. Blanc, E. Manzano, and J.L. Vilchez, Multifocus HDR VIS/NIR hyperspectral imaging and its application to works of art, Opt. Express 27, 11323 (2019).
P. Knipe, K. Eremin, M. Walton, A. Babini, and G. Rayner, Materials and techniques of Islamic manuscripts, Herit. Sci. 6, 55 (2018).
Industrial Applications
Jingli Wang, Jingxiang Gao, Stacking ensemble learning algorithm based rapid inverse modelling of copper grade using imaging spectral data, Chemometrics and Intelligent Laboratory Systems 257, 105308 (2025).
Mukesh Kumar Singh, Subrata Hait, Atul Thakur, Hyperspectral imaging-based classification of post-consumer thermoplastics for plastics recycling using artificial neural network, Process Safety and Environmental Protection 179, 593-602 (2023).
Shuhei Watanabe, Multi-angle measurement device for analysis of coating appearances, Color Research and Appllications 47(1), 27-39 (2022).
Mukesh Chander, Microbial Production and Applications of Pigments, International Research Journal of Engineering and Technology (IRJET) 09(09), 366-375 (2022).
C. Nansen, K. Singh, A. Mian, B.J. Allison, C.W. Simmons, Using hyperspectral imaging to characterize consistency of coffee brands and their respective roasting classes, J. Food Eng. 190, 34-39 (2016).
Physics & Engineering
Zhang, Zheyu & Zhong, Hao & Avramidis, Stavros & Wu, Shuangshuang & Lin, Wenshu & Li, Yaoxiang, Transfer learning for predicting wood density of different tree species: calibration transfer from portable NIR spectrometer to hyperspectral imaging, Wood Science and Technology 59:19, link (2025).
Yasser H. El-Sharkawy, Integrated Optical Coherence Tomography and Hyperspectral Imaging for Automated Structural Health Monitoring of Carbon Fibre Aircraft Structures, Journal of Nondestructive Evaluation 44, 7 (2025).
Alexander E. Siemenn, Eunice Aissi, Fang Sheng, et al., Using scalable computer vision to automate high-throughput semiconductor characterization, Nature Communications 15, 4654 (2024).
C Lukashin, G Kopp, T Jackson, R Swanson, CJ Young
, ARCSTONE InVEST: Calibration of Lunar Spectral Reflectance from Space
, ESTO InVEST-20-001 , Presentation (2024).
James W. Dillon, Christopher P. Donahue, Evan N. Schehrer, and Kevin D. Hammonds, Evaluating sensitivity of optical snow grain size retrievals to radiative transfer models, shape parameters, and inversion techniques, AGUsphere Preprint, Text (2024).
Thomas Pascarella Watson, Kevin McKenzie, Aaron Robinson, Kyle Renshaw, Ron Driggers, Eddie L. Jacobs, and Joseph Conroy, Evaluation of Aerial Real-time RX Anomaly Detection, Proceedings of SPIE - The International Society for Optical Engineering 12519, 125190Q (2023).
Hans Courrier, Rand Swanson, Constantine Lukashin, Christine Buleri, John Carvo, Michael Cooney, Warren Davis, Alexander Halterman, Alan Hoskins, Trevor Jackson, Mike Kehoe, Greg Kopp, Thuan Nguyen, Noah Ryan, Carlos Roithmayr, Paul Smith, Mike Stebbins, Thomas Stone, Cindy Young, ARCSTONE: calibration of lunar spectral reflectance from space. Prototype instrument concept, analysis, and results, Journal of Applied Remote Sensing 17(4), 044508 (2023).
Chunmao Zhu and Yugo Kanaya, Eliminating the interference of water for direct sensing of submerged plastics using hyperspectral near-infrared imager, Nature - Scientific Reports 13, 15991 (2023).
Marc Vermeulen, Alicia McGeachy, Bingjie Xu, Henry Chopp, Aggelos Katsaggelos, Rebecca Meyers, Matthias Alfeld and Marc Walton, XRFast and new software package for processing of MA-XRF datasets using machine learning, Journal of Analytical Atomic Spectrometry 37, 2130-2143 (2022).
L. Logan and J. Shaw, Measuring the polarization response of a VNIR hyperspectral imager, Proc. SPIE 11412, 2558257 (2020).
R.C. Swanson, W.S. Kirk, G.C. Dodge, M. Kehoe, and C. Smith, Anamorphic imaging spectrometers, Proc. SPIE 1098005, 2515641 (2019).
C. Nansen, Penetration and scattering: Two optical phenomena to consider when applying proximal remote sensing technologies to objects, PLoS ONE 13, e0204579 (2018).
E. Pouyet, N. Rohani, A.K. Katsaggelos, O. Cossairt and M. Walton, Innovative data reduction and visualization strategy for hyperspectral imaging datasets using t-SNE approach, Pure Appl. Chem. 90, 493 (2018).
C.I. Zhao, B. Qi, and C. Nansen, Use of local fuzzy variance to extract the scattered regions of spatial stray light influence in hyperspectral images, Optik 124, 6696 (2013).
C.I. Zhao, B. Qi, and C. Nansen, Use of local fuzzy variance to extract the scattered regions of spatial stray light influence in hyperspectral images, Optik 124, 6696 (2013).
C. Nansen, Robustness of analyses of imaging data, Optics Express 19, 16 (2011).
B. Qi, C. Zhao, E. Youn, and C. Nansen, Use of weighting algorithms to improve traditional support vector machine based classifications of reflectance data, Optics Express 19, 27 (2011).
C. Nansen, N. Abidi, A.J. Sidumo, and A.H. Gharalari, Using Spatial Structure Analysis of Hyperspectral Imaging Data and Fourier Transformed Infrared Analysis to Determine Bi, Remote Sensing 2, 908 (2010).
P.W. Nugent, J.A. Shaw, M.R. Kehoe, C.W. Smith, T.S. Moon, and R.C. Swanson, Measuring the modulation transfer function of an imaging spectrometer with rooflines of opportunity, Optical Engineering 49, 103201 (2010).
P.W. Nugent, J.A. Shaw, M.R. Kehoe, C.W. Smith, T.S. Moon, and R.C. Swanson, Measuring the MTF of imaging spectrometers at infinite focus with roofline images, Proc. SPIE 6661, 1 (2007).
Precision Agriculture: Crop Management
Jordan McBreen, Md Ali Babar, Diego Jarquin, Yiannis Ampatzidis, Naeem Khan, Sudip Kunwar, Janam Prabhat Acharya, Samuel Adewale, Gina Brown-Guedira, Enhancing genomic-based forward prediction accuracy in wheat by integrating UAV-derived hyperspectral and environmental data with machine learning under heat-stressed environments, The Plant Genome 18, e20554 (2025).
Jha, Gaurav, Debangshi, Udit, Palla, Sindhu, Nazrul, Farshina, Dey, Sourajit, Dutta, Wri, and Bansal, Sangeeta, Precision Agriculture Technologies for Climate-Resiliency and Water Resource Management, Book Chapter 15, link (2025).
Damian Oswald, Alireza Pourreza, Momtanu Chakraborty, Sat Darshan S. Khalsa, and Patrick H. Brown, 3D radiative transfer modeling of almond canopy for nitrogen estimation by hyperspectral imaging, Precision Agriculture 26(12), link (2025).
Michael B. Farrar, Helen M. Wallace, Iman Tahmasbian, Catherine M. Yule, Peter K. Dunn, Shahla Hosseini Bai, Rapid assessment of soil carbon and nutrients following application of organic amendments, Catena 223, 106928 (2023).
Juncheng Ma, Binhui Liu, Lin Ji, Zhicheng Zhu, Yongfeng Wu, Weihua Jiao, Field-scale yield prediction of winter wheat under different irrigation regimes based on dynamic fusion of multimodal UAV imagery, International Journal of Applied Earth Observation and Geoinformation 118, 103292 (2023).
Longzhe Quan, Zhaoxia Lou, Xiaolan Lv, Deng Sun, Fulin Xia, Hailong Li, Wenfeng Sun, Multimodal remote sensing application for weed competition time series analysis in maize farmland ecosystems, Journal of Environmental Management 344, 118376 (2023).
Wijayanti Nurul Khotimah, Mohammed Bennamoun, Farid Boussaid, Lian Xu, David Edwards, Ferdous Sohel, MCE-ST: Classifying crop stress using hyperspectral data with a multiscale conformer encoder and spectral-based tokens, International Journal of Applied Earth Observation and Geoinformation 118, 103286 (2023).
Chiranjibi Poudyal, Hardev Sandhu, Yiannis Ampatzidis, Dennis Calvin Odero, Orlando Coto Arbelo, Ronald H. Cherry, Lucas Fideles Costa, Prediction of morpho-physiological traits in sugarcane using aerial imagery and machine learning, Smart Agricultural Technology 3, 100104 (2023).
Haiyang Zhang, Yao Zhang, Kaidi Liu, Shu Lan, Tinyao Gao, Minzan Li, Winter wheat yield prediction using integrated Landsat 8 and Sentinel-2 vegetation index time-series data and machine learning algorithms, Computers and Electronics in Agriculture 213, 108250 (2023).
Zongpeng Li , Zhen Chen , Qian Cheng , Fuyi Duan , Ruixiu Sui, Xiuqiao Huang, and Honggang Xu
, UAV-Based Hyperspectral and Ensemble Machine Learning for Predicting Yield in Winter Wheat
, Agronomy 12(1), 202 (2022).
Lucas Costa, Jordan McBreen, Yiannis Ampatzidis, Jia Guo, Mostafa Reisi Gahrooei, Md Ali Babar
, Using UAV-based hyperspectral imaging and functional regression to assist in predicting grain yield and related traits in wheat under heat-related stress environments for the purpose of stable yielding genotypes, Precision Agriculture 23, 622-642 (2022).
Hu Y, Wang Z, Li X, Li L, Wang X, Wei Y., Nondestructive Classification of Maize Moldy Seeds by Hyperspectral Imaging and Optimal Machine Learning Algorithms, Sensors 22(16), 6064 (2022).
Li, Zongpeng and Chen, Zhen and Cheng, Qian and Duan, Fuyi and Sui, Ruixiu and Huang, Xiuqiao and Xu, Honggang, UAV-Based Hyperspectral and Ensemble Machine Learning for Predicting Yield in Winter Wheat, Agronomy 12(1), 202 (2022).
Mónica Pineda and Matilde Barón , Health Status of Oilseed Rape Plants Grown under Potential Future Climatic Conditions Assessed by Invasive and Non-Invasive Techniques, Agronomy 12(8), 1845 (2022).
Bowen Niu, Quanlong Feng, Boan Chen, Cong Ou, Yiming Liu, Jianyu Yanga, HSI-TransUNet: A transformer based semantic segmentation model for crop mapping from UAV hyperspectral imagery, Computers and Electronics in Agriculture 201, 107297 (2022).
Costa, L., McBreen, J., Ampatzidis, Y. et al., Using UAV-based hyperspectral imaging and functional regression to assist in predicting grain yield and related traits in wheat under heat-related stress environments for the purpose of stable yielding genotypes, Precision Agriculture 23, 622-642 (2022).
Ali Missaoui, Sergio Bernardes, Holly Wright, Back in the numbers game: High throughput phenotyping of biomass yield in perennial forage crops with multiple harvests, North American Plant Phenotyping Network November 1, Preprint (2022).
Luís Guilherme Teixeira Crusiol, Liang Sun, Zheng Sun, Ruiqing Chen, Yongfeng Wu, Juncheng Ma, and Chenxi Song, In-Season Monitoring of Maize Leaf Water Content Using
Ground-Based and UAV-Based Hyperspectral Data, Sustainablitity 14, 9039 (2022).
W. Yang, T. Nigon, Z. Hao, G.D. Paiao, F.G. Fernandez, D. Mulla, and C. Yang, Estimation of corn yield based on hyperspectral imagery and convolutional neural network, Comp. Elec. in Ag. 184, 106092 (2021).
Z. Yang, J. Tian, K. Feng, X. Gong, and J. Liu, Application of a hyperspectral imaging system to quantify leaf-scale chlorophyll, nitrogen and chlorophyll fluorescence parameters in grapevine, Plant Phys. and Biochem. 166, 723 (2021).
Y. Zhang, J. Hui, Q. Qin, Y. Sun, T. Zhang, H. Sun, and M. Li, Transfer-learning-based approach for leaf chlorophyll content estimation of winter wheat from hyperspectral data, Remote Sens. Env. 267, 112724 (2021).
S. Yang, L. Hu, H. Wu, H. Ren, H. Qiao, P. Li, and W. Fan, Integration of Crop Growth Model and Random Forest for Winter Wheat Yield Estimation From UAV Hyperspectral Imagery, IEEE J. Selected Topics Appl. Earth Obs. Remote Sens. 14, 6253 (2021).
T.J. Nigon, et al., Prediction of Early Season Nitrogen Uptake in Maize Using High-Resolution Aerial Hyperspectral Imagery, Remote Sens. 12, 12081234 (2020).
K. Zhu, et al., Remotely sensed canopy resistance model for analyzing the stomatal behavior of environmentally-stressed winter wheat, ISPRS J. Phot. Remote Sens. 168, 197 (2020).
P. Fu, K. Meacham-Hensold, M.H. Siebers, and C.J. Bernacci, The inverse relationship between solar-induced fluorescence yield and photosynthetic capacity: benefits for field phenotyping, J. Experimental Botany Dec, 537 (2020).
A. Moghimi, C. Yang, J.A. Anderson, Aerial hyperspectral imagery and deep neural networks for high-throughput yield phenotyping in wheat, eprint arXiv:1906.09666 , arXiv: 09666 (2019).
S. Yang, L. Hu, H. Wu, W. Fan, and H. Ren, Estimation Model of Winter Wheat Yield Based on Uav Hyperspectral Data, IGARSS 2019 - 2019 IEEE International Geoscience and Remote Sensing Symposium , 7212 (2019).
X. Ji'An, C. HongXin, Y. YuWang, Z. WeiXin, W. Qian, X. Lei, G. DaoKuo, Z. WenYu, K. YaQi, and H. Bo, Detection of waterlogging stress based on hyperspectral images of oilseed rape leaves (Brassica napus L.), Computers and Electronics in Agriculture 159, 59 (2019).
B. Scherrer, J. Sheppard, P. Jha, J.A. Shaw, Hyperspectral imaging and neural networks to classify herbicide-resistant weeds, J. of Appl. Remote Sens. 13(4), 044516 (2019).
P.W. Nugent, J.A. Shaw, P. Jha, B. Scherrer, A. Donelick, and V. Kumar, Discrimination of herbicide-resistant kochia with hyperspectral imaging, J. of Appl. Remote Sens. 12(1), 016037 (2018).
Y. Huang, M.A. Lee, V .K. Nandula, and K.N. Reddy, Hyperspectral Imaging for Differentiating Glyphosate-Resistant and Glyphosate-Susceptible Italian Ryegrass, Am. J. Plant Sci. 9, 1467 (2018).
M. Kanning, I. Kühling, D. Trautz, and T. Jarmer, High-Resolution UAV-Based Hyperspectral Imagery for LAI and Chlorophyll Estimations from Wheat for Yield Prediction, Remote Sensing 10, 2000 (2018).
A. Moghimi, C. Yang, M.E. Miller, S.F. Kianian, and P.M. Marchetto, A Novel Approach to Assess Salt Stress Tolerance in Wheat Using Hyperspectral Imaging, Front. Plant Sci. 24, 01182 (2018).
A. Moghimi, C. Yang, and P.M. Marchetto, Ensemble Feature Selection for Plant Phenotyping: A Journey From Hyperspectral to Multispectral Imaging, IEEE Access 6, 2872801 (2018).
S. Gutiérrez, J. Fernández-Novales, M.P. Diago and J. Tardaguila, On-The-Go Hyperspectral Imaging Under Field Conditions and Machine Learning for the Classification of Grapevine Varieties, Front. Plant Sci. 9, 01102 (2018).
M. Morin, R. Lawrence, K. Repasky, T. Sterling, C. McCann, and S. Powell, Agreement analysis and spatial sensitivity of multispectral and hyperspectral sensors in detecting vegetation stress at , J. of Appl. Remote Sens. 11(4) , 046025 (2017).
M.A. Lee, Y. Huang, V.K. Nandula, and K.N. Reddy, Differentiating glyphosate-resistant and glyphosate-sensitive Italian ryegrass using hyperspectral imagery, Proc. SPIE 9108, (2014).
K.N. Reddy, Y. Huang, M.A., Lee, V.K. Nandula, R.S. Fletcher, S.J. Thomson and F. Zhao, Glyphosate-resistant and glyphosate-susceptible Palmer amaranth (Amaranthus palmeri S. Wats.): hyperspectral reflectance, Pest Management Science , (2014).
C. Nansen, Use of Variogram Parameters in Analysis of Hyperspectral Imaging Data Acquired from Dual-Stressed Crop Leaves, Remote Sensing 4, 180 (2012).
C. Nansen, A.J. Sidumo, and S. Capareda, Vairogram analysis of hyperspectral data to characterize the impact of biotic and abiotic stress of maize plans and to e, Applied Spectroscopy 64, 6 (2010).
Precision Agriculture: Pest & Disease Management
Hyoseok Lee, William M. Wintermantel, John T. Trumble, Christian Nansen, Timing matters: remotely sensed vegetation greenness can predict insect vector migration and therefore outbreaks of curly top disease, Journal of Pest Science 98, 607–617 (2025).
Patrice Savi, Samantha Hall, Maria Hernandez, Anil Mantri, Daniel Kliebenstein, and Christian Nansen, Effects of timed LED regimes on tomato plant traits, performance of two-spotted spider mites, and predatory mites (Phytoseiulus persimilis), Pest Management Science 81(4), link (2025).
Jean-Frédéric Guay, William Champagne-Cauchon, Valérie Fournier, and Conrad Cloutier, Wild host fruit–niche diversity of Drosophila suzukii in lowbush blueberry agroecosystems in Saguenay-Lac-Saint-Jean, Québec, Canada, The Canadian Entomologist 155(e2), 1-20 (2023).
Jaafar Abdulridha, An Min, Matthew N. Rouse, Shahryar Kianian, Volkan Isler, and Ce Yang, Evaluation of Stem Rust Disease in Wheat Fields by Drone Hyperspectral Imaging, Sensors 23 (8), 4154 (2023).
R Ganesh Babu and C Chellaswamy, Different stages of disease detection in squash plant based on machine learning, Journal of Biosciences 47, 9 (2022).
Iost Filho, Fernando Henrique and de Bastos Pazini, Juliano and de Medeiros, André Dantas and Rosalen, David Luciano and Yamamoto, Pedro Takao, Assessment of Injury by Four Major Pests in Soybean Plants Using Hyperspectral Proximal Imaging, Agronomy 12(7), 1516 (2022).
Myongkyoon Yang, Physiological Disorder Diagnosis of Plant Leaves Based on Full-Spectrum Hyperspectral Images with Convolutional Neural Network, Horticulturae 8(9), 854 (2022).
María Belén Bainotti, Aplicación de técnicas de imágenes hiperespectrales en el infrarrojo cercano para la determinación de contaminación fungica y deoxinivalenol en trigo, Máster en Gestión e Innovación en la Industria Alimentaria , Master Thesis (2020).
Jaafar Abdulridha, Yiannis Ampatzidis, Jawwad Qureshi, and Pamela Roberts, Laboratory and UAV-Based Identification and Classification of Tomato Yellow Leaf Curl, Bacterial Spot, and Target Spot Diseases in Tomato Utilizing Hyperspectral Imaging and Machine Learning, Remote Sensing 12(17), 2732 (2020).
J. Abdulridha, O. Batuman, and Y. Ampatzidis, UAV-Based Remote Sensing Technique to Detect Citrus Canker Disease Utilizing Hyperspectral Imaging and Machine Learning, Remote Sensing 11, 1373 (2019).
K. Nagasubramanian1, S. Jones, A. K. Singh, S. S., A. Singh, and B. Ganapathysubramanian, Plant disease identification using explainable 3D deep learning on hyperspectral images, Plant Methods 15, (2019).
J. Zhang, Y. Huang, K.N. Reddy, and B. Wang, Assessing crop damage from dicamba on non-dicamba-tolerant soybean by hyperspectral imaging through machine learning, Pest Manag. Sci. 75, 3260 (2019).
Jaafar Naser Abdulridha, Yiannis Ampatzidis, Sri Charan Kakarla, and Pamela D. Roberts
, Detection of target spot and bacterial spot diseases in tomato using UAV-based and benchtop-based hyperspectral imaging techniques, Precision Agriculture 21, 955 - 978 (2019).
C. Nansen and M. R. Strand, Proximal Remote Sensing to Non-destructively Detect and Diagnose Physiological Responses by Host Insect Larvae to Parasitism, Front. Physiol. 9, 01716 (2018).
C. Nansen, X. Zhang, N. Aryamanesh, and G. Yan, Use of variogram analysis to classify field peas with and without internal defects caused by weevil infestation, J. Food Eng. 123, 17 (2014).
C. Nansen, A.J. Sidumo, X. Martini, K. Stefanova, and J.D. Roberts, Reflectance-based assessment of spider mite "bio-response" to maize leaves and plant potassium content in different irri, Computers and Electronics in Agriculture 97, 21 (2013).
C. Nansen, S. Prager, B. Qi, X. Martini, M. Lewis, and K. Vaugn, Using Hyperspectral Imaging in ZC Research, Proc. 11th Annual SCRI Zebra Chip Reporting Session , 70 (2011).
S. Jay, R. Lawrence, K. Repasky, and C. Keith, Invasive species mapping using low cost hyperspectral imagery, ASPRS Annual Conference, Baltimore MD , (2009).
C. Nansen, T. Macedo, R. Swanson, and D.K. Weaver, Use of spatial structure analysis of hyperspectral data cubes for detection of insect-induced stress in wheat plants, International Journal of Remote Sensing 30, 2447 (2009).
Hyperspectral Imaging: General
Zhang, Zhengxin, and Lixue Zhu, Review on Unmanned Aerial Vehicle Remote Sensing: Platforms, Sensors, Data Processing Methods, and Applications, Drones 7(6), 398 (2023).
Agronomy
M. Vahidi, S. Shafian, W. H. Frame, Precision Soil Moisture Monitoring Through Drone-Based Hyperspectral Imaging and PCA-Driven Machine Learning, Sensors 25, 782 (2025).
Huimin Qi, Ang Chen, Xiuchun Yang, and Xiaoyu Xing, Estimation of crude protein content in natural pasture grass using unmanned aerial vehicle hyperspectral data, Computers and Electronics in Agriculture 229, 109714 (2025).
S. Faisal, M. P. -L. Ooi, S. K. Abeysekera, Y. -C. Kuang and D. Fletcher, Roadmap for Measurement and Applications: Uncertainty Quantification and Visualization for Optimal Decision-Making in Hyperspectral Imaging-Based Precision Agriculture, IEEE Instrumentation & Measurement Magazine 28(1), 23-32 (2025).
Nagarajan S․, Maria Merin Antony, Murukeshan Vadakke Matham, Early and accurate nutrient deficiency detection in hydroponic crops using ensemble machine learning and hyperspectral imaging
, 11, 100952 (2025).
Abdulridha, J.; Min, A.; Rouse, M.N.; Kianian, S.; Isler, V.; Yang, C., Evaluation of Stem Rust Disease in Wheat Fields by Drone Hyperspectral Imaging, Sensors 23, 41-54 (2023).
Yan, Y.; Yang, J.; Li, B.; Qin, C.; Ji, W.; Xu, Y.; Huang, Y. , High-Resolution Mapping of Soil Organic Matter at the Field Scale Using UAV Hyperspectral Images with a Small Calibration Dataset, Remote Sensing 15(5), 1433 (2023).
Medical
Ramy Abdlaty, Mohamed A. Abbass, and Ahmed M. Awadallah, Toward near real-time precise supervision of radiofrequency ablation for liver fibrosis using hyperspectral imaging, Spectrochimica Acta Part A: Molecular and Biomolecular Spectroscopy 336, 125994 (2025).
Mohamed Hisham Aref, Sanzhar Korganbayev, Ibrahim H. Aboughaleb, Abdallah Abdelkader Hussein, Mohamed A. Abbass, Ramy Abdlaty, Yasser M. Sabry, Paola Saccomandi, Abou-Bakr M. Youssef , Custom Hyperspectral Imaging System Reveals Unique Spectral Signatures of Heart, Kidney, and Liver Tissues, Spectrochimica Acta Part A: Molecular and Biomolecular Spectroscopy 305, 123363 (2024).
Yasser H. El-Sharkawy, Sherif Elbasuney, Sara M. Radwan, Non-invasive diffused reflected/transmitted signature accompanied with hyperspectral imaging for breast cancer early diagnosis, Optics & Laser Technology 169, 110151 (2024).
Yasser H. El-Sharkawy, Automated hyperspectral imaging for non-invasive characterization of human eye vasculature: A potential tool for ocular vascular evaluation, Experimental Eye Research 240, 109792 (2024).
Wayne S. Holmes; Melanie Po-Leen Ooi; Sanush Abeysekera; Ye Chow Kuang; Ray Simpkin; Manu Caddie, On machine learning methods to estimate cannabidiolic acid content of Cannabis sativa L. from near-infrared hyperspectral imaging, 2023 IEEE International Instrumentation and Measurement Technology Conference (I2MTC) , 01-06 (2023).
Yasser H. El-Sharkawy, Development of a custom optical imaging system for non-invasive monitoring and delineation of lower limb varicose veins using hyperspectral imaging and quantitative phase analysis, Photodiagnosis and Photodynamic Therapy 44, 103808 (2023).
Hafid, A.; Difallah, S.; Alves, C.; Abdullah, S.; Folke, M.; Lindén, M.; Kristoffersson, A., State of the Art of Non-Invasive Technologies for Bladder Monitoring: A Scoping Review, Sensors 23(5), 2758 (2023).
Airborne Hyperspectral Remote Sensing
Jose Ricardo Lima Pinto, Caio Vinicius Gomes Pinhalb, Odair Aparecido Fernandes, and David Luciano Rosalen, Optimal flight height and spectral indices for detecting insect injury in peanut crops using UAS, International Journal of Remote Sensing --, 1-15 (2025).
Luyu Shuai, Zhiyong Li, Ziao Chen, Detao Luo, Jiong Mu, A research review on deep learning combined with hyperspectral Imaging in multiscale agricultural sensing, Computers and Electronics in Agriculture 217, 108577 (2024).
Yeniu Mickey Wang, Bertram Ostendorf, Vinay Pagay, Evaluating the potential of high-resolution hyperspectral UAV imagery for grapevine viral disease detection in Australian vineyards, International Journal of Applied Earth Observation and Geoinformation 130, 103876 (2024).
Ang Chen, Cong Xu, Min Zhang, Jian Guo, Xiaoyu Xing, Dong Yang, Bin Xu, Xiuchun Yang, Cross-scale mapping of above-ground biomass and shrub dominance by integrating UAV and satellite data in temperate grassland, Remote Sensing of Environment 304, 114024 (2024).
A. U. G. Sankararao, P. Rajalakshmi and S. Choudhary, Machine Learning-Based Ensemble Band Selection for Early Water Stress Identification in Groundnut Canopy Using UAV-Based Hyperspectral Imaging, IEEE Geoscience and Remote Sensing Letters 20, 1-5 (2023).
Agriculture & Food Technology
Ban, S.; Tian, M.; Hu, D.; Xu, M.; Yuan, T.; Zheng, X.; Li, L.; Wei, S., Evaluation and Early Detection of Downy Mildew of Lettuce Using Hyperspectral Imagery, Agriculture 15, 444 (2025).
Sanaeifar A, Yang C, Min A, Jones CR, Michaels TE, Krueger QJ, Barnes R, Velte TJ, Noninvasive Early Detection of Nutrient Deficiencies in Greenhouse-Grown Industrial Hemp Using Hyperspectral Imaging, Remote Sensing 16(1), 187 (2024).
Zhang, X., Vinatzer, B.A. & Li, S, Hyperspectral imaging analysis for early detection of tomato bacterial leaf spot disease, Nature Scientific Reports 14, 27666 (2024).
Tahmasbian, I., McMillan, M.N., Kok, J. et al., Underwater hyperspectral imaging technology has potential to differentiate and monitor scallop populations, Reviews in Fish Biology and Fisheries 34, 371–383 (2024).
Sandra Marín-San Román, María Paz Diago, Juan Fernández-Novales, Cristina Cebrián-Tarancón, M. Rosario Salinas, Teresa Garde-Cerdán, Use of the hyperspectral imaging to estimate the volatile composition of Tempranillo grape berries during ripening, Scientia Horticulturae 337, 113537 (2024).
Bernat Borràs-Vallverdú, Sonia Marín, Vicente Sanchis, Ferran Gatius, Antonio J. Ramos, NIR-HSI as a tool to predict deoxynivalenol and fumonisins in maize kernels: a step forward in preventing mycotoxin contamination, Journal of the Science of Food and Agriculture 104, 5495-5503 (2024).
Tony Chuquizuta, Segundo G. Chavez, Alberto Claudio Miano, Marta Castro-Giraldez, Pedro J. Fito, Hubert Arteaga, Wilson Castro, Determination of hydration kinetic of pinto beans: A hyperspectral images application, Measurement: Food 14, 100161 (2024).
J. Muñoz-Postigo, E.M. Valero, M.A. Martínez-Domingo, F.J. Lara, J.L. Nieves, J. Romero, J. Hernández-Andrés, Band selection pipeline for maturity stage classification in bell peppers: From full spectrum to simulated camera data, Journal of Food Engineering 365, 111824 (2024).
Savi, P.J., Mantri, A., Khodaverdi, H. et al., Indirect effects of plasma-activated water irrigation on Tetranychus urticae populations, Journal of Pest Science 2024, Full Text (2024).
Seongmin Park, Myongkyoon Yang, Dong Gyun Yim, Cheorun Jo, Ghiseok Kim, VIS/NIR hyperspectral imaging with artificial neural networks to evaluate the content of thiobarbituric acid reactive substances in beef muscle, Journal of Food Engineering 350, 111500 (2023).
Song, Q., Gao, X., Song, Y. et al., Estimation and mapping of soil texture content based on unmanned aerial vehicle hyperspectral imaging, Nature - Scientific Reports 13, 14097 (2023).
De Silva, A.L.; Trueman, S.J.; Kämper, W.; Wallace, H.M.; Nichols, J.; Hosseini Bai, S. , Hyperspectral Imaging of Adaxial and Abaxial Leaf Surfaces as a Predictor of Macadamia Crop Nutrition, Plants 12(3), 558 (2023).
N. Settouti, O. Assainova, N. A. Saab, and M. El Bouz, Automated Hyperspectral Apple Variety Identification Based on Patch-wise Classification, Optica Sensing Congress 2023 (AIS, FTS, HISE, Sensors, ES), Technical Digest Series (Optica Publishing Group, 2023) , paper JW2A.28 ().
Contact Us
Click below and our hyperspectral experts will contact you soon.
Complete Hyperspectral Imaging Solutions
Contact
Resonon Inc.123 Commercial Drive
Bozeman, MT 59715 USA