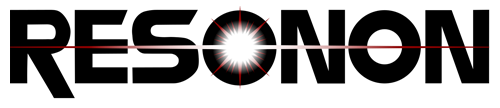
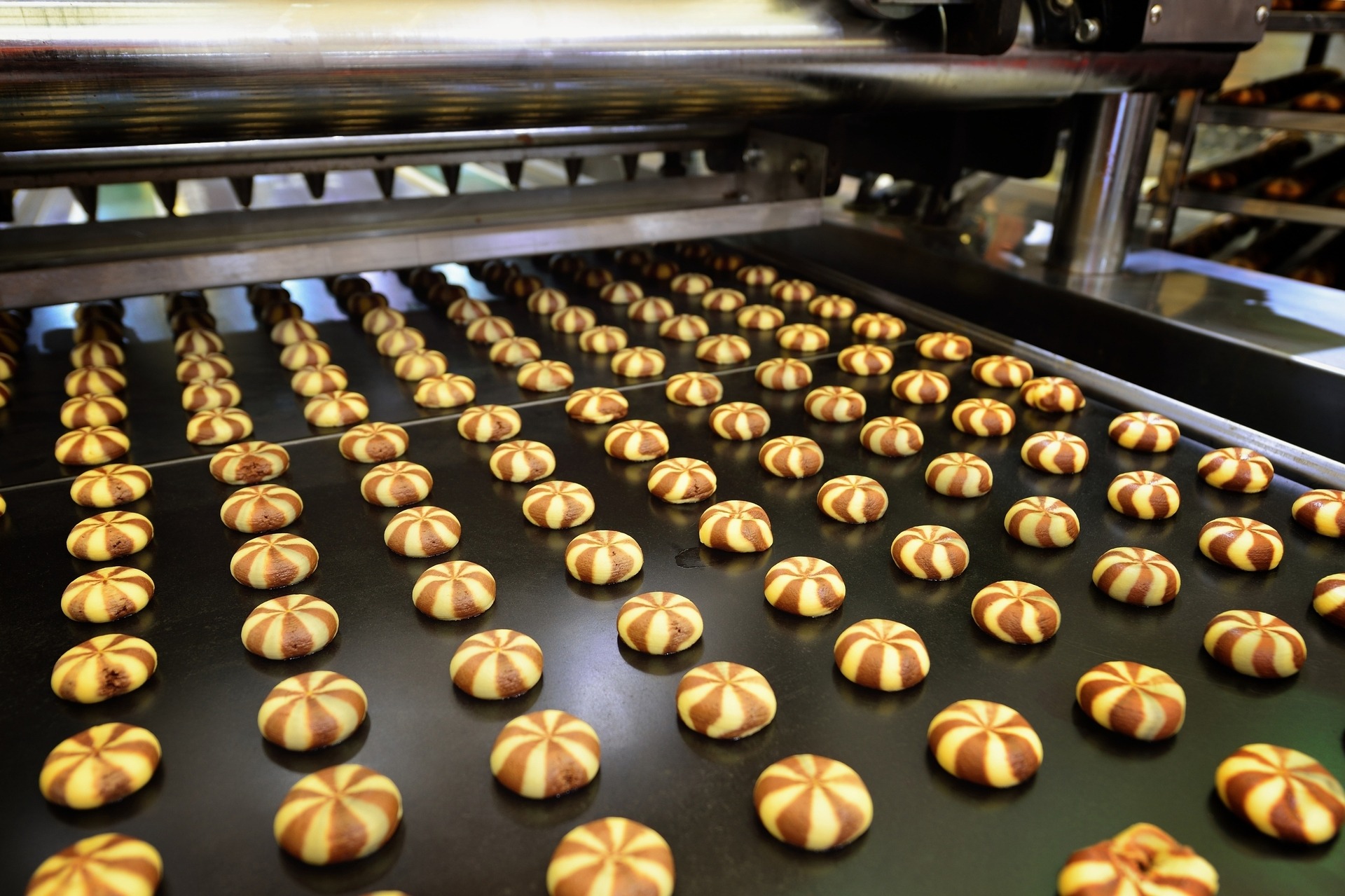
Getting Started with Hyperspectral Machine Vision Systems
By Mike Stebbins, COO & Optomechanical Engineer - January 10, 2024
Hyperspectral machine vision is useful in scenarios that
call for automatic inspection, analysis, and action on the factory floor, such
as automated sorting. Automated sorters typically use hyperspectral imaging to
determine one of two types of information:
- Classification: Category prediction (e.g., acceptable vs. not acceptable, product vs. foreign material, specific type of plastic, bruised vs. unbruised fruit).
- Regression: Quantity prediction (e.g., ripeness, moisture content, percentage of a material in a mixed group, deviation from a nominal value such as product color).
Hyperspectral machine vision systems collect spectral data
from the objects under inspection and then compute the classification or
regression on a per-pixel or per-object basis. Depending on the application, the
results might show information such as defects in the product, mixture ratios,
or debris interspersed among good product.
The results obtained can be translated to meet the input requirements of the factory system, from triggering a subsequent process to alerting an operator to an issue. For example, the system can provide specific coordinates for a picking robot or air jet sorter that will separate the classified products.
Moreover, Resonon’s machine vision software is
easy to use.
See the video above for a short demonstration.
The software’s algorithms learn to detect acceptable and
out-of-spec products using training data. The training data are efficiently assembled,
and multiple algorithms can be tested for optimal performance. By applying
different models, you can determine a solution that meets your application-specific
requirements.
How Shape, Texture, Color, and Orientation Impact Hyperspectral Machine Vision System Outputs
Color difference is another way of saying spectral change. Spectral
differences, even those too small to be detected by the human eye, are what hyperspectral imaging systems use to
differentiate between objects. Object shape statistics (e.g. area, perimeter,
orientation) can also be calculated with Resonon’s RVS software, but these
statistics do not provide the primary information used by the hyperspectral
machine vision system to make predictions.
Product spectral variation and texture generally create
challenges for color-sensitive machine vision systems. A hyperspectral machine
vision system can overcome this challenge by ensuring that the training data
for the classification model include samples encompassing all the spectral
variation and texture that the system will encounter in production. In some
cases, product texture can even be an asset that improves classification accuracy
in a hyperspectral system.
More Questions
Review our extensive FAQ section on the Machine Vision page for more information on Resonon’s machine vision
system.
Resonon’s machine vision experts are also happy to discuss the details
of your application to help you determine whether hyperspectral machine vision is
suitable.
Contact us
Contact usTechnical
Signal-to-Noise Ratio (SNR) in Hyperspectral CamerasDecember 8, 2023
Basics
An Introduction to Hyperspectral ImagingOctober 4, 2023
Contact Us
Click below and our hyperspectral experts will contact you soon.
Complete Hyperspectral Imaging Solutions
Contact
Resonon Inc.123 Commercial Drive
Bozeman, MT 59715 USA