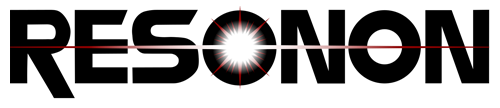
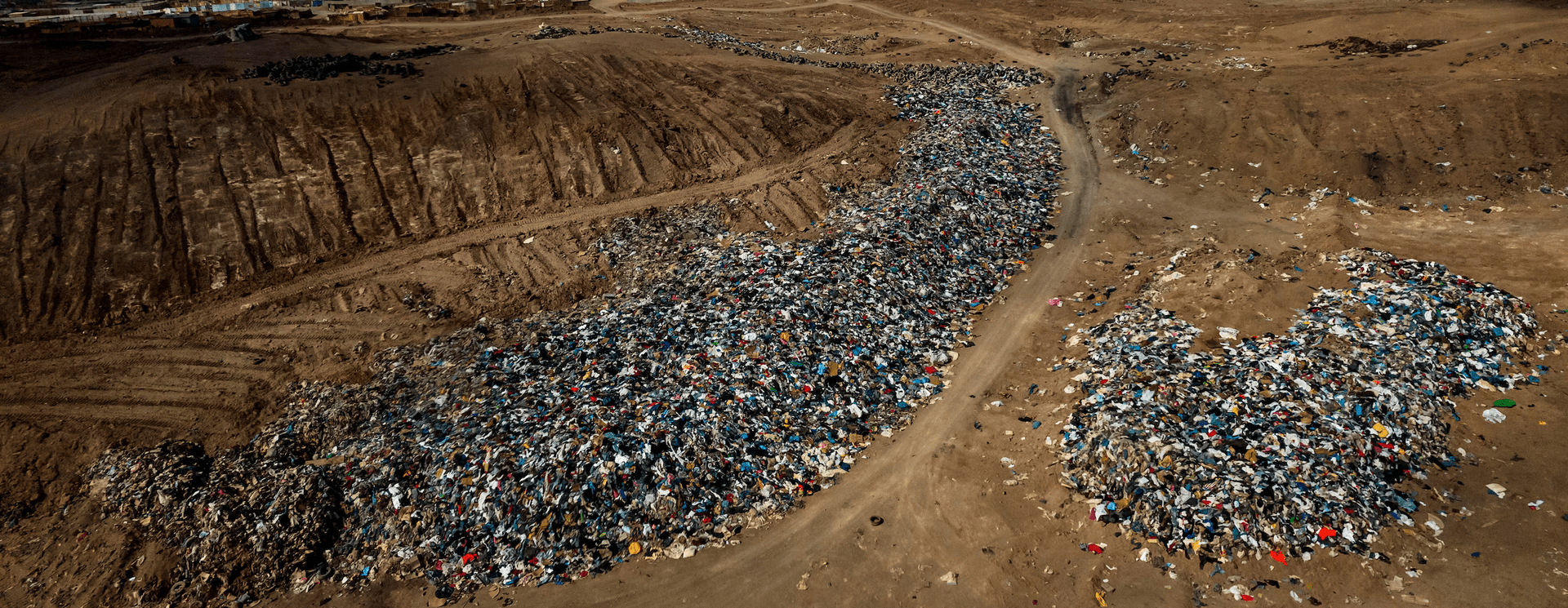
Using Hyperspectral Imaging to Sort Textiles
92M Tons
Textiles into landfills annually (global)10%
Textile production contribution of global carbon emissions
Textiles into landfills annually (global)10%
Textile production contribution of global carbon emissions
Challenge
According to the U.S. Environmental Protection Agency, 17 million
tons of textile were generated in the US in 2018. Two and a half million tons
were recycled, three million tons were combusted for energy, and eleven million
tons were sent to the landfill1. Thus, about 65% of waste textiles
end up in landfills.
Textile waste is increasingly recognized as a significant
environmental problem that currently does not have clear solutions. In 2020,
the EU adopted a new circular economy plan2. Part of this plan
focuses on textiles, with a goal of all textile products placed on the EU
market being long-lived and recyclable by 2030. The commission is also
proposing targets for recycling of textile waste and supports a legislative
target for textile recycling and reuse3. It is a multi-pronged and
incremental approach that also includes regulating the manufacturing industry4.
Recycling will be a key component of reducing textile-related environmental
impacts and it requires dependable sorting technology.
Recycling Solution
Our internal feasibility study shows that hyperspectral
imaging provides sufficient contrast to sort most textiles based on their
spectral signature in the NIR spectral range (from 900 to 1700 nm), mostly
irrespective of their visible color. Therefore, hyperspectral imaging has the
potential to be a crucial technology for sorting textiles for recycling.
Application Overview
We scanned two dozen textile samples of natural, synthetic,
and blended fibers. We then refined several data pre-processing steps and chose
classification algorithms to optimize the system’s ability to distinguish
between various textiles.
After model training, we successfully classified various
chopped textile fibers and a few full clothing items in real-time on our
experimental conveyor station. See the video below.
Equipment Used
We used Resonon’s Real-time Vision System (RVS)
software and a Pika IR to scan
textile samples. Scanning was completed with our SpectralSight™ System using two 4-fixture halogen lighting units.
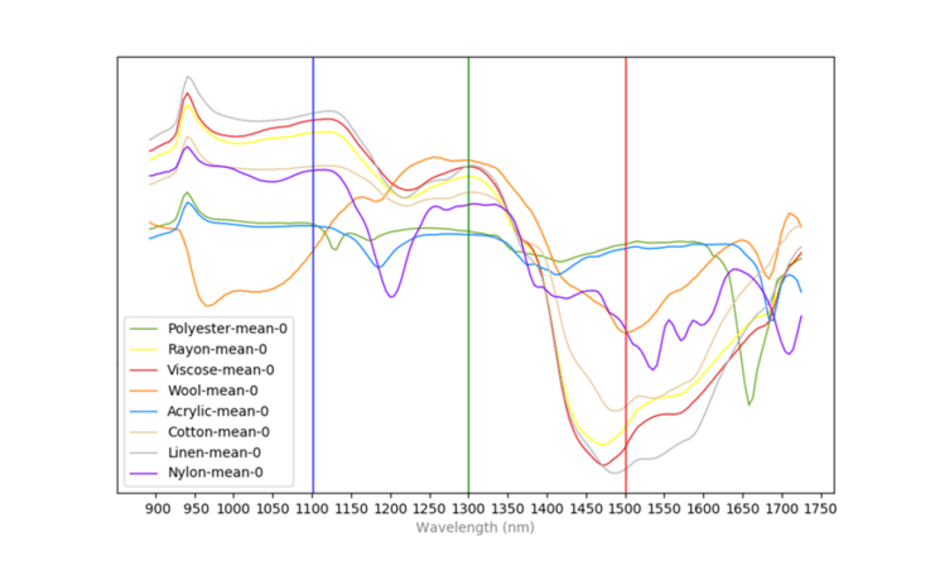
Results
Synthetic fibers are the easiest to distinguish due to their
well-defined and unique chemical bonds, which lead to characteristic spectral
signatures that are readily classified by machine learning algorithms.
Natural fibers such as cotton and linen are made of similar
cellulose building blocks. Their spectral signatures are distinct from the
synthetic fibers but more similar with respect to each other.
There are several hybrid textiles, such as viscose and
rayon, that are chemically processed fibers from natural sources. Since they
are made of natural fibers (wood or other plant-based fibers) their spectral
signatures are closer to those of cotton and linen.
Wool has a unique spectral signature with a broad dip in
reflectance around 1000 nm that was not present in any other fibers. As a
result, wool is easily sorted from other fabrics.
The video below shows RVS classifying chopped textile fibers
and a few full clothing items in real-time.
Despite having never seen the clothing items prior to this
test, the system unambiguously classifies Polyester shorts and wool socks, and
the polyester band on the wool socks.
Some textile datacubes are available on downloads.resonon.com. You can
download a free copy of Spectronon™ and the datacubes there.
For this case study, securing an adequate training sample
set was more time-consuming than the study itself. It took less than 30 minutes
to scan samples, train a classification model, and start classifying textiles
with SpectralSight™.
To further advance this preliminary textile sorting system,
the next step would be to train the RVS software with more samples,
representative of the wide variety of existing textiles including blends like cotton/polyester
and wool/acrylic.
Nonetheless, based on the success of this study, it is safe
to conclude that textile sorting devices that utilize hyperspectral imaging
will be robust, accurate, and reliable.
Contact Resonon
to discuss your application and how we can help you solve challenges with
hyperspectral imaging.
References
- https://www.epa.gov/facts-and-figures-about-materials-waste-and-recycling/textiles-material-specific-data
- https://environment.ec.europa.eu/strategy/textiles-strategy_en
- https://environment.ec.europa.eu/document/download/74126c90-5cbf-46d0-ab6b-60878644b395_en?filename=COM_2022_141_1_EN_ACT_part1_v8.pdf
- https://www.reuters.com/sustainability/eu-wants-all-textile-waste-rules-place-by-2028-commissioner-2023-06-27/
- https://earth.org/statistics-about-fast-fashion-waste/
- https://www.europarl.europa.eu/topics/en/article/20201208STO93327/the-impact-of-textile-production-and-waste-on-the-environment-infographics
- https://www.nationalgeographic.com/environment/article/chile-fashion-pollution (source of Header image)
Contact us
Contact usMore Case Studies
Contact Us
Click below and our hyperspectral experts will contact you soon.
Complete Hyperspectral Imaging Solutions
Contact
Resonon Inc.123 Commercial Drive
Bozeman, MT 59715 USA